How an open recruitment for researchers—and criticism too—led to impressive achievements
—Dr. Ueda, please tell us about your current research activities.
In brief, I research machine learning technology. When I first started on this path more than 20 years ago, I was involved in core theoretical research focused on machine learning in the field of artificial intelligence (AI), which has recently taken on a broader meaning. Over time, I expanded my research into applications and practical uses of machine learning in conjunction with big data and the Internet of Things (IoT).
Machine learning technology consists of various types of learning methods. One such method is pattern recognition as used in character recognition. This type of learning involves, for example, teaching a computer how to identify a cat by declaring “This is a cat” when presenting a photograph of a cat. Another type of learning is clustering as a basic process of data analysis, which involves judging on one’s own what is common and different between a cat and some other entity.
However, in keeping with today’s information society, we can say that machine learning is more than just pattern recognition; it is a technology for extracting latent information behind data. Statistical learning, which is my area of specialization, treats the data generation process as a probabilistic model. It learns the model from observation data and extracts latent information via this model (Fig. 1).
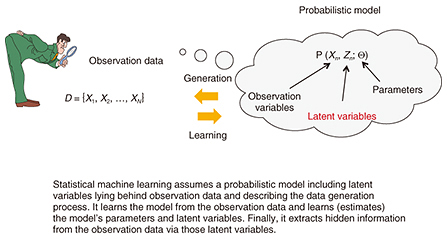
Fig. 1. Statistical machine learning technology.
Presently, as director of the Machine Learning and Data Science Center, I am promoting research and development (R&D) that will enable machine learning technology within the NTT AI technology brand corevo® to be put to good use in the real world. Specifically, this is R&D on a system for smoothing out the flows of people and traffic by predicting congestion arising from unforeseen circumstances using a technology called ambient intelligence and providing that information beforehand in real time. This system could be used to provide high-speed evacuation guidance in the event of a disaster.
The technologies used here, and in particular, crowd navigation technology that can respond to unforeseen circumstances, constitute a challenging research theme since they have yet to be used in the real world. We have named this kind of analysis technology targeting such spatio-temporal data typified by people and traffic flows spatio-temporal multidimensional collective data analysis (Fig. 2) [1].
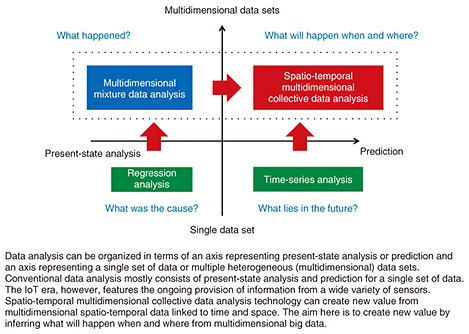
Fig. 2. Spatio-temporal multidimensional collective data analysis.
With conventional technology, it has been difficult to make near-future predictions that specify time and space such as when and where some type of event such as congestion will occur. In contrast, the spatio-temporal multidimensional collective data analysis that we are developing can make a near-future prediction of the period (time) and place (space) of an event that will take place several hours into the future from big data generated sometime in the past. Additionally, as the IoT era gathers momentum, our aim is to optimize the flow of information gathered from a massive number of diverse types of sensors through repeated observation, analysis, prediction, and provision (guidance) in real time. This optimization process should prove useful in preventing congestion itself, controlling communication traffic at the time of a sports or music event, controlling traffic according to the degree of congestion, and guiding visitors in shopping malls, airports, and other places.
—What led you to take on this research theme?
The catalyst was an open recruitment for researchers within the NTT laboratories more than 20 years ago. At that time, in the middle of the AI boom, I applied for a position in AI research at NTT Communication Science Laboratories. To give some background here, it had been decided to set up a basic research laboratory at Keihanna Science City in Kyoto, which generated a demand for young researchers in all of those laboratories. I was initially involved in basic research in the field of computer vision focusing on the theme of image analysis from line drawings, but my main interest at that time was statistical learning theory. This research of image processing that I had undertaken focused on process, that is, a method of cleaning up a picture or clarifying its contour. However, what I really wanted to know was not the shape of the picture but what the target object actually was. In other words, I was more concerned with achieving “understanding” or “recognition.” To this end, I resolved to move to the field of machine learning, which I thought would provide me with more theoretical opportunities, and I applied for a transfer with my research plan in hand.
Machine learning began in the 1970s as a field within AI, and neural networks boomed in the 1980s as a type of machine learning applicable to a variety of application fields. This boom, however, lasted only a few years, and perhaps as a reaction to this short-lived technology, machine learning went on to evolve into somewhat low-key mathematical research called learning theory. Against this background, I took up research at NTT Communication Science Laboratories, but two years later, a turning point happened in my career; my research in the field of vector quantization resulted in awards that put me on the path to overseas research stays.
Then, having launched a new research theme, I took up the challenge of submitting a paper to Neural Information Processing Systems (NIPS), a prestigious conference in machine learning known to be highly competitive. That paper was the first one by a Japanese researcher to be accepted, and it provided me with an opportunity to present my research results (Fig. 3) [2]. I think my motivation behind this challenge was partially in response to the criticism I received from the then laboratory director, who had said to me, “Your group is doing nothing meaningful or useful.”
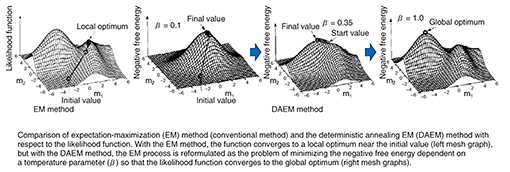
Fig. 3. Overview of method reported in NIPS paper.
Then, in the aftermath of the NIPS paper, I received an invitation from Dr. Geoffrey Hinton, a world authority on neural networks, to visit the University of Toronto in Canada, and I ended up spending about a month there. Dr. Hinton told me he didn’t really care for statistics and mathematics very much, and contrary to my expectations, I did not receive direct research guidance from him. But thinking that I had to produce some results, I became absorbed in my work. Indeed, I was in crisis mode, as I had to accept the fact that my connection with Dr. Hinton would probably come to an end if I could not generate any results in that one month. I dived into my research forgetting to eat and sleep in an attempt to produce something one way or another, and in the end, the results that I achieved became the seeds of research that was again selected for publication by NIPS.
At that time, I wasn’t sure what the results themselves would be useful for, but after Dr. Hinton evaluated them again, I was invited to a new research laboratory at University College London in the United Kingdom that he had founded, and I remember being called the “first visitor” in a lighthearted manner.
I then expanded my research into the field of speech recognition and took up technology for achieving a simpler and more efficient speech recognition model. This research was a joint effort with speech recognition researchers at that research laboratory, but the results achieved were evaluated by a number of parties and received various awards. The core of these results, however, consisted of basic theoretical research. On reflection, I feel that the sum total of the research on theoretical systems that I carried out in my younger days laid the foundation for my present research. The rebellious spirit of my senior researchers and the harsh criticism of the laboratory director at that time, the challenges presented to me by my group leaders, the existence of NTT Communication Science Laboratories, and the environment made available to me by Dr. Hinton have all contributed to what I am today.
The ability to produce your research theme is the key
There is much joy in making the most of opportunities and delivering results
—So your encounter with a pioneering researcher and your presentation at a highly competitive international conference were filled with drama!
Because of these experiences, my research activities expanded greatly from what had been work in a somewhat narrow field. The second half of the 1990s saw a trend toward investing in machine learning, and the multiplex topic classification technology for text that I developed was put to use in the goo portal site operated by NTT Resonant for classifying news articles and performing other functions. Then in 2005, I met Dr. Masaru Kitsuregawa, an authority on data engineering, and became involved in the Funding Program for World-Leading Innovative R&D on Science and Technology (FIRST) of the Cabinet Office, Government of Japan, in the role of a sub-theme leader in Dr. Kitsuregawa’s research project.
In this program, 30 core researchers were selected from all research fields in Japan, and Dr. Kitsuregawa was the sole researcher selected from the field of information engineering. His project theme was the development of an ultra-high-speed database, and I was entrusted with the sub-theme of determining in what fields such a database, if achieved, could be applied (Fig. 4) [3]. Together with more than ten co-researchers, I focused on big data analysis in the field of healthcare. In particular, I took up the challenge of automatically recognizing nursing actions and analyzing the effects of those actions on patients, working with doctors at Kyushu University Hospital.
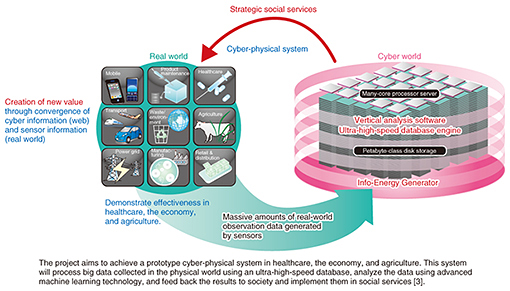
Fig. 4. Overview of Dr. Kitsuregawa’s project.
Achieving automatic recognition of nursing actions was a very difficult endeavor. Although broad, obvious actions such as running could be easily recognized, relatively calm and understated actions such as patient interviewing and drawing blood presented a problem. That is, under the constraints of automatic recognition using just a few acceleration sensors affixed to a nurse so as not to hamper daily nursing tasks, the application of existing machine learning techniques could not achieve sufficient accuracy here for practical implementation. Doctors at the hospital would make disparaging comments like “Is this the best machine learning can do?” Additionally, as it appeared that no journal paper was in the offering under these circumstances, the collaborating doctors distanced themselves from the research one after another.
Nevertheless, as I was the one responsible for this research, I persisted with this theme for another year and a half. In the end, I succeeded in creating a meta-learning method that I used to perfect a high-accuracy automatic recognition technique. I then went on to analyze data covering about 9 million nursing actions and to analyze the effects of nursing intervention on the admitting and releasing of patients. This big data analysis was the first of its kind in the world, and it received high marks in the final FIRST evaluation for opening up a new path in healthcare through the use of information and communication technology.
More recently, I have come to be involved in research related to cosmic physics. This research involves using the Subaru Telescope on the summit of Mauna Kea on the island of Hawaii and is aimed at estimating and analyzing the distance to supernovae about 10 billion light years away and constructing a history of universe expansion. The researchers here need to instantly and automatically identify supernovae and collect data on them, and I have helped meet this need using machine learning technology.
Further classification of supernovae types is just getting under way. At the University of Tokyo, the Kavli Institute for the Physics and Mathematics of the Universe (Kavli IPMU) [4]—the client of this joint research—received praise for this new research approach using machine learning and consequently became the first Japanese research institution to get observation time on the Hubble Space Telescope, which has been providing valuable astronomical data of historic proportions. In this way, Kavli IPMU has been able to obtain even more data on supernovae, for which I am grateful.
This means that we can now perform world-leading research by combining the wide-field Subaru Telescope and the high-accuracy Hubble Space Telescope, which is a very satisfying outcome.
—Surely you have gone where no one has gone before!
I would not make such a claim—I think it’s more an outcome of good fortune. However, I would say that I have been successful in finding promising new themes and taking up the challenge of trying to solve difficult research and analysis problems. This process begins with the people one encounters and what kind of joint research one pursues, and looking back, I believe that making an effort to produce results and not simply giving up is how I have been able to create new opportunities.
For example, when given the chance to analyze nursing actions from Dr. Kitsuregawa, I certainly did not want to waste that valuable opportunity, and knowing that there would not be another chance if I produced nothing of merit, I felt a sense of urgency. I refused to give up and went on to create the meta-learning method, working one step at a time in a low-key manner for about two years.
Remembering the sense of excitement and reading as many journal papers as possible with great intent—steady effort opens up the future
—What would you say to young researchers?
To each of our young researchers, I would say, “Charge ahead in your chosen theme with all of your heart and soul.” Many things in life like something someone says or current social trends can weaken your resolve, but don’t lose focus—hold steady and be true to yourself. Don’t become demoralized or run away in the face of harsh comments. At the same time, don’t let the burden of responsibility make you continuously anxious; face the problem with a sense of balance. When the crunch comes, you may forget to sleep and eat as you look for a solution, but it’s okay to take a break too.
In basic research, the probability of a hit is 1 out of 1000 in extreme cases, so a lot of time and patience are needed. Whether one can endure such frustration is the key to success. A superficial paper, though easy to write, cannot be very meaningful or influential. Like all of us, I have taken on difficult problems that I worked tirelessly on with my share of ups and downs. When I first took up the challenge of submitting a paper to NIPS and received the mail notifying me of its acceptance, I wanted to cry out in a loud voice from the bottom of my heart. I still can’t forget that feeling of joy, and I ask all of you as well not to forget what such a sense of accomplishment feels like.
—What then, should researchers focus on when they are young?
At present, I’m involved in applied research making use of my research achievements to date. I am convinced that cultivating basic technology in a dedicated manner when I was young was extremely important in making this happen. I believe that the basics play an important role not just in research overall but in the life of the individual researcher too. For example, a technology called reinforcement learning that was first devised about 30 years ago is used in the AlphaGo go playing program that has recently become a topic of conversation. Back then, no one thought that this technology would be used in a program for playing go, but as it turned out, AlphaGo could not have been achieved without it. So I would like young researchers who have much time ahead of them to work at building such a foundation. Without accumulating basic technologies, nothing new will come into view.
—So accumulating results is important for young researchers having much time ahead of them.
Yes, and in addition, journal papers are useful in establishing a network of contacts. In my younger days, publishing papers was a motivating force behind my research, but nowadays, I pursue research that, if anything, can have a direct impact on society. To this end, I feel that encountering brilliant researchers to form partnerships for collaborative work is essential, and to get such topnotch researchers interested in your work, it is important, after all, to focus intently on writing good papers and getting noticed. Compared with the past, it is easy to search for papers in today’s environment, which makes it easier to obtain evaluations from outside the laboratory than from inside.
It is also important to hold discussions with a variety of researchers to generate new ideas. Each researcher has a problem that he or she is dealing with, and simply talking about your respective problems and worries may provide a hint to solving your own research problem. Here, getting your partner to open up requires that you build a trustworthy relationship, so when there are partners in dissimilar fields, I make it a point to visit with those partners often. I myself am an elemental-technology techie, that is, a “hammer-and-saw” tool techie! And to provide tools with value, it is important to talk beforehand with a specialist in that area on what kind of house we should construct. There is no need to worry about each other’s status here—we should form relationships frequently to learn new things from others.
“What” is what’s necessary for research, while “how” is what’s important in a paper—top-level researchers focus on “what”
—Dr. Ueda, in closing, what do you see as future issues in machine learning, and what is your personal outlook for the future?
I place much importance on educating young researchers. Since top-level young researchers study an awful lot on their own, I’m always thinking of how I can broaden their research fields and provide them with a stimulating environment rather than simply guiding them in their research. I want to create a space in which they can demonstrate their abilities freely. By the way, machine learning, while heretofore having a somewhat shunned existence, now has wind in its sails, so to speak, as AI comes to be generally accepted. However, this boom too will one day subside, so I want to establish an environment in which young researchers can continue their research at that time. This is the mission of a senior researcher.
I would also like to contribute to solving problems that researchers in a variety of fields are dealing with. At this point in my research career, I feel that there is more value in making concrete contributions than in writing papers. For example, I want to see ambient intelligence and spatio-temporal prediction that I talked about earlier to continue developing as far as verification experiments and actual operation. This type of research on social infrastructure cannot proceed by one company alone. Partnerships must be formed with related companies, and progress must be steadily made while making adjustments.
Of course, this is easy to say but difficult to achieve in practice. If any party pursues only its own interests, the collaborative effort will break down, so a balance between cooperation and competition must be achieved. Writing research papers is not the only work of a researcher. I believe that experienced researchers and research leaders are required to look at things from their partners’ point of view and to achieve even better technology.
Although “how” is of course important in the case of a paper, “what” is important in the activities of an experienced researcher. A specialist is interested in “how,” but the impact of technology on society is “what.” Research that can clearly explain what has been developed and what purpose it can serve in society is research at its best. Actually, it’s only recently that I became aware of this. This is because elemental-technology techies are a species of researchers who are often interested only in “how.” In addition, a small amount of difference in “how” has nearly no value. Furthermore, if a technology is not usable in many areas, that technology can be only halfway satisfying. At the risk of repeating myself, if a researcher cannot produce results in the form of “what,” that research will likely have no impact on society.
Services and products such as Uber, Pokémon Go, and AlphaGo that have swept society are all combinations of existing technologies; they have not made any contributions in the form of new technologies. However, beating the world’s champion go player even with a combination of existing technologies is an outstanding achievement that can have a great impact. If researchers themselves in an information society dominated by the service industry can produce value and high-impact results, certainly the quality of research will change significantly.
Today’s young researchers worry that their papers may go unnoticed even if the papers are well written. For this reason, I would like to provide extensive guidance to young researchers on the ability to produce value and results. I point out one more time that communicating with researchers in a variety of fields is a great way of refining one’s ability to produce results as a researcher.
References
[1] | F. Naya, M. Miyamoto, and N. Ueda, “Optimal Crowd Navigation via Spatio-temporal Multidimensional Collective Data Analysis,” NTT Technical Review, Vol. 15, No. 9, 2017.
https://www.ntt-review.jp/archive/ntttechnical.php?contents=ntr201709fa5.html |
[2] | N. Ueda and R. Nakano, “Deterministic Annealing Variant of the EM Algorithm,” Neural Information Processing Systems 7 (NIPS7), MIT Press, Cambridge, MA, USA, pp. 545–552, 1995. |
[3] | Website of the “Development of the Fastest Database Engine for the Era of Very Large Database and Experiment and Evaluation of Strategic Social Services Enabled by the Database Engine” project.
http://www.tkl.iis.u-tokyo.ac.jp/FIRST/outline.en.html |
[4] | Website of Kavli IPMU,
http://www.ipmu.jp/en/news-information |
Trademark notes
All brand names, product names, and company names that appear in this article are trademarks or registered trademarks of their respective owners.