
You need Adobe Reader 7.0 or later in order to read PDF files on this site.
If Adobe Reader is not installed on your computer, click the button below and go to the download site.
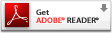
|
External Awards
Research Paper Award
Winner: Kimihiro Mizutani, Takeru Inoue, Toru Mano, and Osamu Akashi, NTT Network Innovation Laboratories; Satoshi Matsuura, Tokyo Institute of Technology; Kazutoshi Fujikawa, Nara Institute of Science and Technology Date: September 19, 2017
Organization: Japan Society for Software Science and Technology (JSSST)
For “Stable Load Balancing with Overlapping ID-space Management in Range-based Structured Overlay Networks.”
Published as: K. Mizutani, T. Inoue, T. Mano, O. Akashi, S. Matsuura, and K. Fujikawa, “Stable Load Balancing with Overlapping ID-space Management in Range-based Structured Overlay Networks,” Computer Software, JSSST, Vol. 32, No. 3, pp. 101–110, Aug. 2015.
Best Paper Award
Winner: Takashi Miyamura, NTT Network Service Systems Laboratories; Akira Misawa, Chitose Institute of Science and Technology (CIST); Jun-ichi Kani, NTT Access Network Service Systems Laboratories Date: September 27, 2017
Organization: The Asia-Pacific Network Operations and Management Symposium (APNOMS) 2017
For “Design of Optical Aggregation Network with Carrier Edge Functions Virtualization.”
Published as: T. Miyamura, A. Misawa, and J. Kani, “Design of Optical Aggregation Network with Carrier Edge Functions Virtualization,” IEICE Tech. Rep., Vol. 117, No. 186, PN2017-26, pp. 69–74, Aug. 2017.
ACC TOKYO CREATIVITY AWARDS
Interactive Category
New Technology Award
Winner: Katsuhiko Kawazoe, Tomoyuki Kanekiyo, and Shinji Fukatsu, NTT Service Innovation Laboratory Group; Ryuji Kubozono, Akihito Akutsu, Kenichi Minami, Akira Ono, Takashi Miyatake, Koichi Furukado, Hidenobu Nagata, Hiromu Miyashita, Hirokazu Kakinuma, Mariko Yamaguchi, Tetsuya Yamaguchi, Yosihide Tonomura, Takako Sato, Hideaki Takada, Kimitaka Tsutsumi, Yumi Kikuchi, Toshiharu Morizumi, Shingo Kinoshita, Manabu Motegi, Kyoko Hashiguchi, and Kenya Suzuki, NTT Service Evolution Laboratories; Tatsuya Fujii, Naohiro Kimura, Takahiro Yamaguchi, and Ippei Shake, NTT Network Innovation Laboratories; Soichiro Usui, NTT Research and Development Planning Department Date: September 27, 2017
Organization: All Japan Radio & Television Commercial Confederation (ACC)
For “Kirari! KABUKI.”
An initiative for delivering the glamour of kabuki to the world using state-of-the-art technology. A live kabuki performance staged in Las Vegas, USA, was broadcast in Tokyo, Japan. The footage was reproduced later in a highly immersive manner in Kumamoto, Japan.
Spikes Asia 2017
Innovation: Shortlist (Applied Innovation)
Music: Shortlist (Music Live Experience)
Winner: Shingo Kinoshita, Hiroshi Chigira, and Kenichi Minami, NTT Service Evolution Laboratories; Tomoyuki Kanekiyo, NTT Service Innovation Laboratory Group; Kenya Suzuki, Koji Namba, Masato Ono, Motohiro Makiguchi, Hiromu Miyashita, Hirokazu Kakinuma, Takeru Isaka, Hidenobu Nagata, and Hikaru Takenaka, NTT Service Evolution Laboratories Date: September 29, 2017
Organization: Spikes Asia
For “CYBER TELEPORTATION TOKYO.”
The spatial layout of nine transparent screens, including one double-sided screen, was used to create an engaging and immersive experience made possible through a powerful communication infrastructure. The ultralow latency multimedia synchronized transmission technology over high-speed networks achieved a transfer distance of 6543 miles with zero packet loss using 2K x 5 feeds with 600-ms latency (from Tokyo to Austin, Texas, including 75-ms network latency during the event), and 4K x 13 feeds (tested on maximum transfer size in previous trials), and next generation real-time image extraction technology from any background. We applied our technology to extract in 80 ms human shapes of the artists in Tokyo from a background of choice under various stage lighting conditions and without using conventional green screen technology, then transmitted these isolated images. It was the world debut of a double-sided transparent screen projection to display human form image extractions (double-sided screen provided by Polid Screen).
CHEMINAS Outstanding Research Award
Winner: Tetsuhiko Teshima, Hiroshi Nakashima, Yuko Ueno, Satoshi Sasaki, Calum Henderson, and Shingo Tsukada, NTT Basic Research Laboratories Date: October 5, 2017
Organization: The 36th Chemistry and Micro-Nano Systems conference (CHEMINAS 36)
For “Cell Assembly in Self-folded Multi-layered Soft Films.”
Published as: T. Teshima, H. Nakashima, Y. Ueno, S. Sasaki, C. Henderson, and S. Tsukada, “Cell Assembly in Self-folded Multi-layered Soft Films,” CHEMINAS 36, 3P-26, Kiryu, Gunma, Japan, Oct. 2017.
Papers Published in Technical Journals and Conference Proceedings
Improved Cladding-pumped 32-core Multicore Fiber Amplifier
S. Jain, T. Mizuno, Y. Jung, A. Isoda, K. Shibahara, J. R. Hayes, Y. Sasaki, K. Takenaga, Y. Miyamoto, S. Alam, and D. J. Richardson
Proc. of the 43rd European Conference on Optical Communication (ECOC 2017), Th.2.D.2, Gothenburg, Sweden, September 2017.
We present an improved cladding pumped high-core-count 32-core multicore-fiber amplifier. A gain of >17 dB and average NF (noise figure) of 6.5 dB with <2 dB variation is obtained for –4 dBm input signal power over all cores in the wavelength range 1534 nm–1561 nm.
Crosstalk Analysis of 32-core Dense Space Division Multiplexed System for Higher Order Modulation Formats Using an Integrated Cladding-pumped Amplifier
C. Castro, S. Jain, Y. Jung, E. De Man, S. Calabrò, K. Pulverer, M. Bohn, J. R. Hayes, S. U. Alam, D. J. Richardson, Y. Sasaki, T. Mizuno, K. Shibahara, T. Kobayashi, Y. Miyamoto, T. Morioka, and W. Rosenkranz
Proc. of ECOC 2017, M.2.E.5, Gothenburg, Sweden, September 2017.
We analyse the crosstalk performance of a fully integrated inline amplified 32-core link for 100G quadrature phase-shift keying (QPSK), 150G 8 quadrature amplitude modulation (QAM), 200G 16-QAM, and 250G 32QAM in a recirculating loop. Transmission distances over 1000 km are confirmed for 8-QAM and QPSK channels.
Hybrid Cladding-pumped EDFA/Raman for SDM Transmission Systems Using Core-by-core Gain Control Scheme
T. Mizuno, A. Isoda, K. Shibahara, H. Ono, M. Fukutoku, and Y. Miyamoto
Proc. of ECOC 2017, M.1.E.3, Gothenburg, Sweden, September 2017.
We propose and demonstrate hybrid cladding-pumped/Raman amplification for multicore fiber transmission systems. 46WDM (wavelength division multiplexing) PDM (polarization-division multiplexed)-16QAM signals were transmitted over a 7-core transmission line and Q-factor enhancement is demonstrated using the core-by-core gain control scheme.
50 ch x 250 Gbit/s 32-QAM Transmission over a Fully Integrated 7-core Multicore Link
C. Castro, S. Jain, Y. Jung, E. De Man, S. Calabrò, K. Pulverer, M. Bohn, J. R. Hayes, S. U. Alam, D. J. Richardson, Y. Sasaki, T. Mizuno, Y. Miyamoto, T. Morioka, and W. Rosenkranz
Proc. of ECOC 2017, M.1.E.5, Gothenburg, Sweden, September 2017.
A transmitted distance of 180 km over an integrated multicore link is demonstrated for a C-band 32-QAM WDM system, where the complete usable amplification region of the integrated 7-core amplifiers, supporting 50 channels per core, is exploited.
Adaptive Rates of High-spectral-efficiency WDM/SDM Channels Using PDM-1024-QAM Probabilistic Shaping
H. Hu, M. P. Yankov, F. Da Ros, Y. Amma, Y. Sasaki, T. Mizuno, Y. Miyamoto, M. Galili, S. Forchhammer, L. K. Oxenløwe, and T. Morioka
Proc. of ECOC 2017, Tu.1.D.1, Gothenburg, Sweden, September 2017.
We demonstrate adaptive rates and spectral efficiencies in WDM/SDM (space division multiplexing) transmission using probabilistically shaped PDM-1024-QAM signals, achieving up to 7-Tbit/s data rates per spatial-super-channel and up to 297.8-bit/s/Hz aggregate spectral efficiency using a 30-core fiber on 12.5 and 25-GHz WDM grids.
Recursive Extraction of Modular Structure from Layered Neural Networks Using Variational Bayes Method
C. Watanabe, K. Hiramatsu, and K. Kashino
Proc. of Discovery Science 2017, pp. 207–222, Kyoto, Japan, September 2017.
Deep neural networks have made a substantial contribution to the recognition and prediction of complex data in various fields, such as image processing, speech recognition and bioinformatics. However, it is very difficult to discover knowledge from the inference provided by a neural network, since its internal representation consists of many nonlinear and hierarchical parameters. To solve this problem, an approach has been proposed that extracts a global and simplified structure for a neural network. Although it can successfully detect such a hidden modular structure, its convergence is not sufficiently stable and is vulnerable to the initial parameters. In this paper, we propose a new deep learning algorithm that consists of recursive back propagation, community detection using a variational Bayes, and pruning unnecessary connections. We show that the proposed method can appropriately detect a hidden inference structure and compress a neural network without increasing the generalization error.
↑ TOP
|