1. Introduction
An ideal baseball pitcher can throw a ball to the same location repeatedly. A hallmark of motor skill lies in the ability to carry out actions with both accuracy and precision. While the learning of accurate actions has been revealed by decades of studies, comparatively less is known about how the brain learns to improve movement precision.
Movements are made possible by muscles. The brain sends electrical signals to contract the muscles, which leads to joint motion. Since muscles can only contract, at least two muscles are needed to control a joint. A motion such as moving an elbow from one position to another is composed of a burst in flexor-muscle activity, which accelerates the limb, followed by a burst in extensor activity that slows the elbow down (Fig. 1(a)). In a landmark paper, Harris and Wolpert showed that noise in the muscle’s activity scales with its activation amplitude. Thus, force variability tends to increase with the magnitude of the applied force [1] (Fig. 1(b)). This signal-dependent noise is believed to be a major source of force variability in humans. Another studied showed that sensory noise in vision when localizing the target or final position augments force variability [2]. Others have noticed that noise in planning the duration of the movement can lead to force and movement variability as well [3, 4]. While previous studies have described several sources of noise that contribute to force variability, to the best of our knowledge, no study has investigated how noise in the timing of a burst in muscle activity could lead to force variability. To fill this gap, we explored whether and how volatility in the timing of muscle activity could contribute to force variability.
2. Motor timing as a source of force variability
Day et al. showed that the entire muscle-activity pattern from a single muscle can shift in time when the brain is blasted by an electromagnetic pulse given just before the muscle is activated [5]. The muscle activity’s shape remained unchanged, and only its timing was affected. If the brain has a mechanism that controls the timing of muscle activity independently of its amplitude, then neural noise could cause the muscle to activate earlier or later than the intended time. A crude simulation of the resultant force from mistimed flexor and extensor activity revealed substantial force variability when the timing of each burst fluctuated (Fig. 1(c)). Mistimed muscle activity also shifted the timing of the peak force unlike with signal-dependent noise and baseline noise where only its amplitude was affected. When the brain controls the arm’s force, how much of its variability can be explained by timing volatility?
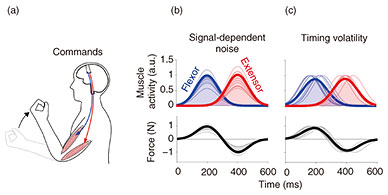
Fig. 1. Force variability during movements as predicted from signal-dependent noise and noise in motor timing.
3. An experiment to observe the arm’s force variability
We asked ten right-handed participants to use their left shoulder to push and pull a robotic handle, the position of which was fixed to prevent motion (Fig. 2(a)). A speaker produced periodic beeps at a frequency of either 3, 3.5, 4, 4.5, 5, or 5.5 Hz. Participants had to use their shoulder to push and pull the handle to hit two targets displayed on a monitor at the designated frequency. During this task, the shoulder’s force and the muscle activity from its flexor and extensor muscles were recorded. The force trajectory from an exemplar participant showed that the force variability grew as the speaker’s frequency increased (Fig. 2(b)). To quantify this relationship, we calculated the force magnitude from each trial and examined how its variability changed as a function of the frequency. Data from all participants revealed that the force variability positively correlated with its frequency (Fig. 2(c)). What source of noise in the muscle activity contributed to this increase in force variability?
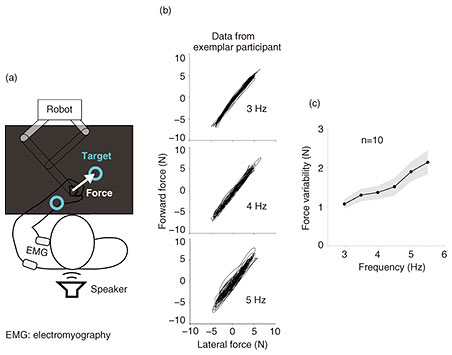
Fig. 2. Experiment to measure the arm’s force variability during rhythmic force production. Force variability increased with frequency.
4. A model of shoulder-force control
The shoulder’s force variability could come from several different sources. To understand their contributions to the final force variability, we constructed a computer model of the shoulder controlled by flexor and extensor muscles that pushed and pulled against a rigid constraint similar as in the experiment. In this model, we considered how the muscle activity was corrupted by three sources of noise. First, a baseline noise changes the entire amplitude of the muscle activity (blue in Fig. 3(a)). Second, signal-dependent noise modulates the amplitude of the muscle activity, effectively scaling its size (red in Fig. 3(a)). Third, the timing of the muscle activity changes from one burst to the next (magenta in Fig. 3(a)). When simulating each burst in muscle activity, these three sources of noise were controlled independently. Noise was resampled for every burst. We used model predictive control (MPC) to optimize the flexor and extensor muscle activity to generate the correct amount of force at the intended frequency [6] (Fig. 3(b)). After this optimization process, noise was added to the muscle activity.
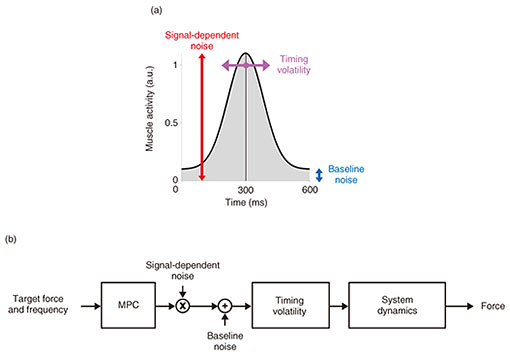
Fig. 3. Computer model of the shoulder to test the source of noise that could explain the empirically observed force variability.
We first determined whether our model could generate muscle activity that resembled those recorded during the experiment. Figure 4(a) shows the filtered muscle activity and force of an exemplar participant producing a force at 5 Hz. When the model was set to produce a force at 5 Hz, the simulated muscle activity and force resembled the empirical data (right of Fig. 4(a)). Using this human-like model, we manipulated the baseline noise, signal-dependent noise, and timing volatility independently to best fit the data from the experiment. Importantly, the amount of baseline noise and timing volatility was kept the same for all frequencies, but signal-dependent noise was increased linearly from 3 to 5.5 Hz since such noise increases with speed [1]. However, the size of the signal-dependent noise was kept below 5% within the empirically observed range [7, 8]. When the noise from one source was manipulated, the other two noise sources were fixed. This enabled us to study the effects of each source of noise independently and assess their ability to explain the data.
Baseline noise only increased the force variability evenly for all frequencies (top of Fig. 4(b)). While signal-dependent noise caused the force variability to increase as a function of the frequency, the predicted force variability underestimated the observed value. Furthermore, signal-dependent noise could not accurately predict the variability in the peak force’s timing (bottom of Fig. 4(b)). When timing volatility was injected into the model, the simulated force’s variability gradually increased with frequency as in the data, and timing volatility predicted the variability in the peak force’s timing more accurately than baseline noise and signal-dependent noise. Thus, while baseline and signal-dependent noise in the muscles affects the amount of force variability and can explain its dependence on frequency, only timing volatility could accurately predict both the force variability and variability in the peak force’s timing.
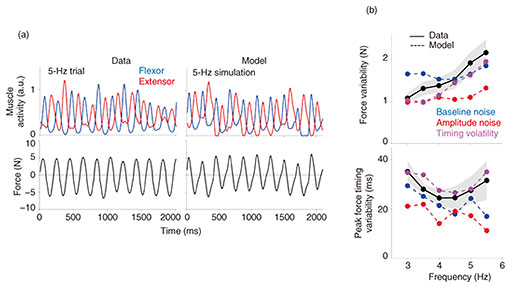
Fig. 4. Computer model of the shoulder produced simulated muscle activity and force that resembled those recorded during the experiment.
Next, we calculated the variability in the timing of the peak force in both the data and model (bottom of Fig. 4(b)). Again, the model with timing volatility was most accurate at explaining the variability in the peak force’s timing. These results suggest that fluctuations in the timing of muscle activity play an important role in determining the variability of the arm’s force.
5. Conclusion
We conducted an experiment and simulation to understand how different sources of noise in muscle activity could affect the variability in the arm’s force. While both baseline and signal-dependent noise play an important role in movement variability, they could not explain how force variability increases as a function of its frequency. The results suggest that timing volatility could be an important factor that sways the variance of the arm’s force. Future research will delve into how timing volatility affects the variability of movements and determine whether the source of variable timing is in the periphery or is more centrally located in the brain.
References
[1] |
C. M. Harris and D. M. Wolpert, “Signal-dependent Noise Determines Motor Planning,” Nature, Vol. 394, No. 6695, pp. 780–784, Aug. 1998.
https://doi.org/10.1038/29528 |
[2] |
C. Ghez, J. Gordon, and M. F. Ghilardi, “Impairments of Reaching Movements in Patients without Proprioception. II. Effects of Visual Information on Accuracy,” J. Neurophysiol., Vol. 73, No. 1, pp. 361–372, Jan. 1995.
https://doi.org/10.1152/jn.1995.73.1.361 |
[3] |
D. E. Meyer, J. E. Smith, and C. E. Wright, “Models for the Speed and Accuracy of Aimed Movements,” Psychol. Rev., Vol. 89, No. 5, pp. 449–482, Sept. 1982.
https://doi.org/10.1037/0033-295X.89.5.449 |
[4] |
R. Osu, K. Morishige, J. Nakanishi, H. Miyamoto, and M. Kawato, “Practice Reduces Task Relevant Variance Modulation and Forms Nominal Trajectory,” Sci. Rep., Vol. 5, Art. no. 17659, Dec. 2015.
https://doi.org/10.1038/srep17659 |
[5] |
B. L. Day, J. C. Rothwell, P. D. Thompson, A. Maertens de Noordhout, K. Nakashima, K. Shannon, and C. D. Marsden, “Delay in the Execution of Voluntary Movement by Electrical or Magnetic Brain Stimulation in Intact Man: Evidence for the Storage of Motor Programs in the Brain,” Brain J. Neurol., Vol. 112, No. 3, pp. 649–663, June 1989.
https://doi.org/10.1093/brain/112.3.649 |
[6] |
A. Takagi, H. Gomi, E. Burdet, and Y. Koike, “A Model Predictive Control Strategy to Regulate Movements and Interactions,” bioRxiv, p. 2022.08.24.505193, Aug. 2022.
https://doi.org/10.1101/2022.08.24.505193 |
[7] |
A. Adam, C. J. De Luca, and Z. Erim, “Hand Dominance and Motor Unit Firing Behavior,” J. Neurophysiol., Vol. 80, No. 3, pp. 1373–1382, Sept. 1998.
https://doi.org/10.1152/jn.1998.80.3.1373 |
[8] |
K. E. Jones, A. F. de C. Hamilton, and D. M. Wolpert, “Sources of Signal-dependent Noise during Isometric Force Production,” J. Neurophysiol., Vol. 88, No. 3, pp. 1533–1544, Sept. 2002.
https://doi.org/10.1152/jn.2002.88.3.1533 |
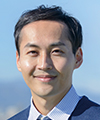 |
- Atsushi Takagi
- Distinguished Researcher, Sensory and Motor Research Group, Human Information Science Laboratory, NTT Communication Science Laboratories.
He received an MSci. in physics and Ph.D. in computational neuroscience from Imperial College London in 2011 and 2016. He was a recipient of the Japan Science and Technology Agency’s PRESTO grant between 2018 and 2022. In 2020, he joined NTT Communication Science Laboratories to study how the brain controls movements. He is a member of the Japanese Society for Motor Control and the Japanese Neural Network Society.
|