
You need Adobe Reader 7.0 or later in order to read PDF files on this site.
If Adobe Reader is not installed on your computer, click the button below and go to the download site.
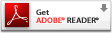
|
Regular Articles
Vol. 23, No. 1, pp. 50–55, Jan. 2025. https://doi.org/10.53829/ntr202501ra1
AI-powered Beamforming for Listening to Moving Talkers
Tsubasa Ochiai, Marc Delcroix, Tomohiro Nakatani,
and Shoko Araki
Abstract
Speech enhancement extracts the voice we want to listen to from the background noise and is essential for speech applications, such as automatic speech recognition, to work effectively in noisy environments. This article introduces a novel beamforming technique that tracks the speaker’s movement and keeps extracting the target speaker’s voice, even when the speaker is moving while talking. Beamforming requires spatial information of the target source and interfering signals such as the direction of arrival. We discuss our previously proposed method to estimate time-varying spatial information by incorporating powerful artificial intelligence technology. This method enables high-performance beamforming even when the target speaker is moving.
Keywords: beamforming, artificial intelligence, moving sources
1. Introduction
Speech processing technology has greatly progressed, and speech interfaces, such as smart speakers, have become widely used in our daily lives. We are constantly immersed in many types of sounds, including ambient noise and interfering speakers. Such interfering signals can degrade the performance of speech processing application such as automatic speech recognition (ASR). To mitigate such degradation, speech interfaces often use multiple microphones (microphone array) and apply array signal processing techniques to suppress the interfering signals and enhance the target sources’ signals.
Beamforming [1] (also called spatial filtering) has been an active research field for several decades and extensively used to design speech enhancement systems for hearing aids [2] and speech interfaces [3]. Beamforming exploits the spatial information about the target and interfering sources and emphasizes the signals coming from a target source direction while suppressing the interfering signals coming from other directions. It plays an important role in developing far-field speech processing application and has been used as the de facto standard front-end in meeting analysis (i.e., far-field speaker diarization and ASR) challenges [4].
Considering realistic situations, such as application to hearing aids or smart speakers, the target and interfering sources may move, e.g., the talkers walk around the room while speaking (see Fig. 1). However, most conventional studies assume a static situation in which the sources do not move within an utterance. Applying such conventional beamformers to dynamic situations in which the sources move results in sub-optimal performance.
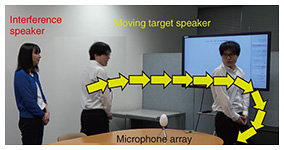
Fig. 1. Assumed scenario in which the target source moves while talking.
To construct effective beamforming filters, accurate estimation of the spatial information (i.e., information on the direction of the target and interfering sources) is essential. However, the spatial information changes at every moment in dynamic situations; thus, the estimation problem of such time-varying spatial information becomes more difficult than that in static situations. We introduce our recent study [5], in which we proposed an estimation method of the spatial information of moving sources by incorporating powerful artificial intelligence (AI) technology. By using developments in the AI field, i.e., attention mechanism [6], our method allows the beamforming to steer its directivity at each time frame toward the position of the moving source, i.e., enabling source tracking.
2. Overview of beamforming
In speech processing, beamforming filters are often designed in the short-time Fourier transform (STFT) domain. Let Yt,ƒ ∈ ℂC be a vector comprising the C-channel STFT coefficients of the observed signal at a time-frequency bin (t,ƒ), which is recorded using a C-channel microphone array and potentially contaminated with ambient noise and interfering speakers. The objective of beamforming is to recover (enhance) the target source signal from such multichannel noisy observation. Given the observed noisy signal Yt,ƒ, beamforming estimates the enhanced signal St,ƒ ∈ ℂ by linearly filtering the observed signal Yt,ƒ with beamforming filter wt,ƒ ∈ ℂC as
where and † denotes the conjugate transpose.
Here, FBF(⋅) denotes the beamforming function [1], which indicates that the beamforming filter wt,ƒ is constructed on the basis of the spatial information (i.e., spatial covariance matrices) of the target and interfering signals respectively. From Eq. (1), the problem of constructing effective beamforming filters is considered the problem of estimating accurate spatial information.
3. AI-powered neural beamforming for moving sources
Beamforming relies on spatial statistics of the target source and interfering signals, which is typically computed by considering the entire observed signal, e.g., 10 seconds long. However, if the source moves, considering the entire signal results in a beamforming filter that does not steer in the correct source direction. We propose instead to estimate the spatial statistics using only the observation that are relevant at a given time, which allows designing a beamforming filter that can track the source movement. Finding which part of observation to use at a given time is challenging because it depends on the way the source moves (e.g., velocity and trajectory). Thus, we design a powerful AI model to estimate which observation to use to obtain optimal beamforming filters.
In our previous study [5], we found that the conventional estimation method of spatial information can be expressed using the following general formulation:
where ν = {S, I}, T denotes the number of time frames, and denotes instantaneous spatial information, which includes spatial information for each time frame.
By accumulating instantaneous statistics with weight coefficients , we can obtain an estimate of spatial information that is statistically more reliable. The weight coefficients indicate the importance of each frame in estimating spatial information at a given time frame t. Equation (2) is analogous to that of the attention mechanism [6], which is the key component of the success of AI technology. Thus, we refer to these weight coefficients as attention weights.
In previous studies, the attention weights for beamforming were determined by heuristic and deterministic rules such as in an online [7] or blockwise [8] manner. However, such simple rules may not be necessarily optimal for handling a variety of moving patterns such as different trajectories/velocities of sources and different ambient noise conditions. To estimate the optimal attention weights in various situations, we thus proposed a neural-network-based attention weight estimation model , where , F denotes the number of frequency bins, and NN(⋅) denotes a neural-network-based function, i.e., self-attention model [6]. On the basis of the supervised learning (data-driven) framework, we train the self-attention model to estimate the attention weights that can extract the target source signals while removing the interfering signals. By incorporating a variety of moving patterns in the training set, the training procedure enables the model to estimate the attention weights that are optimal for tracking the positions of moving sources in various situations.
Figure 2 illustrates the behavior of the attention weights as the target speaker moves. The green and red boxes show examples of attention weights when the target speaker is talking from a direction corresponding to around about 45 and 135 degrees, respectively. The attention weights take high values for time frames where the target speaker is close to the current direction. As seen in the figure, the attention weights change when the target speaker moves, which enables us to track the speaker and estimate reliable spatial information at each time frame.
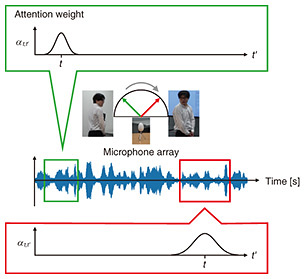
Fig. 2. Schematic image of attention weights’ behavior.
Figure 3 shows the processing flow of our attention-based beamforming method. First, given the observed noisy signal Yt,ƒ, the instantaneous spatial information is computed. Next, the attention weight estimation model computes the attention weights Αν. Then, the spatial information is computed on the basis of the instantaneous spatial information and attention weights by using Eq. (2). Finally, the constructed beamforming filters wt,ƒ are applied to the observed signal, and the enhanced signal St,ƒ is generated using Eq. (1).
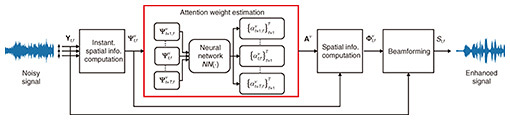
Fig. 3. Processing flow of our attention-based beamforming method.
4. Performance evaluation
We conducted experiments to evaluate the speech enhancement performance of our method. We created an evaluation dataset consisting of simulated moving sources under noisy conditions. For each recording, a single talker is speaking while moving, and the microphone observations are contaminated with ambient noise in public noisy environments such as cafe, street junction, public transportation, and pedestrian area. We used a rectangular microphone array (on a tablet device) with five channels.
Figure 4 shows the objective speech enhancement measures (i.e., signal-to-distortion ratio (SDR) [9]) of the evaluated signals: 1) unprocessed signals, 2) beamformed signals (conventional), and 3) beamformed signals (ours). We observed that our method significantly improved speech enhancement performance compared with the conventional method.
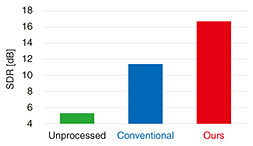
Fig. 4. Evaluation of speech enhancement performance of our and conventional methods. Higher score indicates better performance.
Figure 5 shows an example of the evaluated signals recorded in a real-world environment such as that in Fig. 1. Focusing on the latter part of the waveform of the conventional method, the amplitude of the beamformed signal becomes smaller, which suggests the failure of source tracking (i.e., the beamformer’s directivity is not steered toward the position of the moving source). Our method preserves the amplitude of the target source signal for the entire utterance, which shows the success of source tracking.
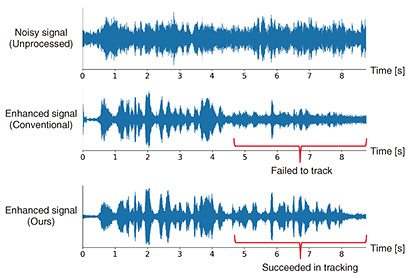
Fig. 5. Example waveforms of evaluated signals.
5. Future perspectives
Our attention-based beamforming method can automatically track a speaker’s movement, enabling a beamforming method to keep listening while a talker is moving. Our method opens new possibilities for speech interfaces, hearing aids, and robots, which could lead to a future in which people and machines can interact more naturally in any situation such as when multiple speakers talk while freely walking around.
We built a demo system using our method and confirmed that it can work for real-world recordings. However, issues need to be addressed before the method can be widely used. For example, our current method incurs high computation costs when using the attention weight estimation model. Thus, an investigation for low-latency processing would be required to allow quick response. In addition, the training stage of our method requires simulating microphone observations of moving sources. Improving the reality of these simulations may be important to further improve performance and make the method more robust to various recording conditions.
References
[1] |
J. Benesty, J. Chen, and Y. Huang, “Microphone Array Signal Processing,” Springer Topics in Signal Processing, Vol. 1, Springer Berlin, Heidelberg, 2008. |
[2] |
S. Doclo, S. Gannot, M. Moonen, and A. Spriet, “Acoustic Beamforming for Hearing Aid Applications,” Handbook on Array Processing and Sensor Networks (eds. S. Haykin and K. R. Liu), pp. 269–302, 2010. |
[3] |
R. Haeb-Umbach, J. Heymann, L. Drude, S. Watanabe, M. Delcroix, and T. Nakatani, “Far-field Automatic Speech Recognition,” Proceedings of the IEEE, Vol. 109, No. 2, pp. 124–148, 2021.
https://doi.org/10.1109/JPROC.2020.3018668 |
[4] |
S. Watanabe, M. Mandel, J. Barker, E. Vincent, A. Arora, X. Chang, S. Khudanpur, V. Manohar, D. Povey, D. Raj, D. Snyder, A. S. Subramanian, J. Trmal, B. B. Yair, C. Boeddeker, Z. Ni, Y. Fujita, S. Horiguchi, N. Kanda, T. Yoshioka, and N. Ryant, “CHiME-6 Challenge: Tackling Multispeaker Speech Recognition for Unsegmented Recordings,” Proc. of 6th International Workshop on Speech Processing in Everyday Environments (CHiME 2020), Virtual, May 2020.
https://doi.org/10.21437/CHiME.2020-1 |
[5] |
T. Ochiai, M. Delcroix, T. Nakatani, and S. Araki, “Mask-based Neural Beamforming for Moving Speakers with Self-attention-based Tracking,” IEEE/ACM Transactions on Audio, Speech, and Language Processing, Vol. 31, pp. 835–848, 2023.
https://doi.org/10.1109/TASLP.2023.3237172 |
[6] |
A. Vaswani, N. Shazeer, N. Parmar, J. Uszkoreit, L. Jones, A. N. Gomez, L. Kaiser, and I. Polosukhin, “Attention Is All You Need,” in Advances in Neural Information Processing Systems, pp. 5998–6008, 2017. |
[7] |
T. Higuchi, N. Ito, T. Yoshioka, and T. Nakatani, “Robust MVDR Beamforming Using Time-frequency Masks for Online/Offline ASR in Noise,” Proc. of the 41st International Conference on Acoustics, Speech and Signal Processing (ICASSP 2016), pp. 5210–5214, 2016.
https://doi.org/10.1109/ICASSP.2016.7472671 |
[8] |
Y. Kubo, T. Nakatani, M. Delcroix, K. Kinoshita, and S. Araki, “Mask-based MVDR Beamformer for Noisy Multisource Environments: Introduction of Time-varying Spatial Covariance Model,” Proc. of the 24th International Conference on Acoustics, Speech and Signal Processing (ICASSP 2019), pp. 6855–6859, 2019.
https://doi.org/10.1109/ICASSP.2019.8683092 |
[9] |
E. Vincent, R. Gribonval, and C. Fevotte, “Performance Measurement in Blind Audio Source Separation,” IEEE Transactions on Audio, Speech, and Language Processing, Vol. 14, No. 4, pp. 1462–1469, 2006.
https://doi.org/10.1109/TSA.2005.858005 |
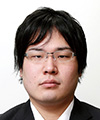 |
- Tsubasa Ochiai
- Research Scientist, Signal Processing Research Group, Media Information Laboratory, NTT Communication Science Laboratories.
He received a B.E., M.E., and Ph.D. from Doshisha University, Kyoto, in 2013, 2015, and 2018. He was a corporative researcher with National Institute of Information and Communications Technology from 2013 to 2018 and research fellow of Japan Society for the Promotion of Science from 2015 to 2018. He has been a researcher at NTT Communication Science Laboratories since 2018. His research interests include speech and spoken language processing such as automatic speech recognition, speech enhancement, array signal processing, and machine learning. He is a member of the Acoustical Society of Japan (ASJ) and the Institute of Electrical and Electronics Engineers (IEEE). He received the Best Student Presentation Award from ASJ in 2014, Student Paper Award from the IEEE Kansai Section in 2016, Awaya Prize Young Researcher Award from ASJ in 2020, Itakura Prize Innovative Young Researcher Award from ASJ in 2021, Best Paper Runner-Up Award from the ACM Multimedia in 2022, and IEEE Signal Processing Society (SPS) Japan Young Author Best Paper Award from IEEE SPS Tokyo Joint Chapter in 2023.
|
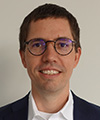 |
- Marc Delcroix
- Distinguished Researcher, Signal Processing Group, Media Information Laboratory, NTT Communication Science Laboratories.
He received an M.Eng. from the Free University of Brussels, Brussels, Belgium, and the Ecole Centrale Paris, Paris, France, in 2003, and Ph.D. from the Graduate School of Information Science and Technology, Hokkaido University, in 2007. He is currently a distinguished researcher at NTT Communication Science Laboratories. He was a research associate at NTT Communication Science Laboratories from 2007 to 2008 and from 2010 to 2012, where he became a permanent research scientist in 2012. He was a visiting lecturer at the Faculty of Science and Engineering, Waseda University, Tokyo, from 2015 to 2018. His research interests include speech and audio processing such as target speech and sound extraction, speech enhancement, robust speech recognition, model adaptation, and speaker diarization. He took an active part in the development of NTT robust speech recognition systems for the REVERB, CHiME 1, and CHiME 3 challenges, which all achieved the best performance results in the tasks. He is a member of the steering committee of the CHiME challenge and member of ASJ. He also served as a member of the IEEE SPS and the Speech and Language Processing Technical Committee from 2018 until 2023, of the organizing committee for the REVERB challenge 2014, the IEEE Automatic Speech Recognition and Understanding Workshop (ASRU) 2017, and the IEEE Spoken Language Technology Workshop (SLT) 2022. He received the 2005 Young Researcher Award from the Kansai Section of ASJ, the 2006 Student Paper Award from the IEEE Kansai Section, the 2006 Sato Paper Award from ASJ, the 2015 IEEE ASRU Best Paper Award Honorable Mention, and the 2016 ASJ Awaya Young Researcher Award.
|
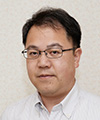 |
- Tomohiro Nakatani
- Senior Distinguished Researcher, Signal Processing Group, Media Information Laboratory, NTT Communication Science Laboratories.
He received a B.E., M.E., and Ph.D. from Kyoto University in 1989, 1991, and 2002. In 2005, he was a visiting scholar at the Georgia Institute of Technology, USA, and from 2008 to 2017, he served as a visiting associate professor in the Department of Media Science at Nagoya University, Japan. Since joining NTT as a researcher in 1991, he has focused on developing audio signal processing technologies for intelligent human-machine interfaces, including dereverberation, denoising, source separation, and robust automatic speech recognition. He served as an associate editor for the IEEE Transactions on Audio, Speech, and Language Processing from 2008 to 2010. He was a member of the IEEE SPS Audio and Acoustics Technical Committee from 2009 to 2014, the IEEE SPS Speech and Language Processing Technical Committee from 2016 to 2021, and the IEEE SPS Fellow Evaluation Committee in 2024. He co-chaired the 2014 REVERB Challenge Workshop and was a general co-chair of IEEE ASRU 2017. He received the 2005 Institute of Electronics, Information and Communication Engineers (IEICE) Best Paper Award, the 2009 ASJ Technical Development Award, the 2012 Japan Audio Society Award, an Honorable Mention for the 2015 IEEE ASRU Best Paper Award, the 2017 Maejima Hisoka Award, and the 2018 International Workshop on Acoustic Echo and Noise Control (IWAENC) Best Paper Award. He is a fellow of IEEE and IEICE and a member of ASJ.
|
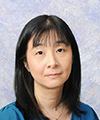 |
- Shoko Araki
- Group Leader and Senior Research Scientist, Signal Processing Group, Media Information Laboratory, NTT Communication Science Laboratories.
She received a B.E. and M.E. from the University of Tokyo in 1998 and 2000 and Ph.D. from Hokkaido University in 2007. Since she joined NTT in 2000, she has been engaged in research on acoustic signal processing, array-signal processing, blind source separation, meeting diarization, and auditory scene analysis. She was a member of the IEEE SPS Audio and Acoustic Signal Processing Technical Committee (2014–2019), a vice chair (2022), and currently serves as its chair (2023–2024). She was a board member of ASJ (2017–2020) and served as vice president of ASJ (2021–2023). She received the 19th Awaya Prize from ASJ in 2001, the Best Paper Award of the IWAENC in 2003, the TELECOM System Technology Award from the Telecommunications Advancement Foundation in 2004 and 2014, the Academic Encouraging Prize from IEICE in 2006, the Itakura Prize Innovative Young Researcher Award from ASJ in 2008, the Commendation for Science and Technology by the Minister of Education, Culture, Sports, Science and Technology, the Young Scientists’ Prize in 2014, IEEE SPS Best Paper Award in 2014, and IEEE ASRU 2015 Best Paper Award Honorable Mention in 2015. She is an IEEE Fellow.
|
↑ TOP
|